In the ever-evolving landscape of clinical trials, artificial intelligence (AI) emerges not merely as a trend but as a beacon of transformative potential. According to GlobalData’s annual industry survey, pharma professionals have consistently heralded AI as a game-changer from 2020 through 2023. Yet, amid this digital revolution, one question looms large: which variant of AI reveals the most promise?
The applications of AI are both vast and intricate, beckoning pharmaceutical companies to adopt a deliberate and value-oriented approach. The emergence of large language models (LLMs), exemplified by the likes of ChatGPT, has captivated global attention due to their remarkable ability to generate coherent text on demand. Yet, this vast creativity carries with it a double-edged sword—while LLMs can conjure seemingly boundless responses, their reliability remains questionable.
Supervised machine learning (ML) offers a cautious yet strategic pathway forward. While LLMs thrive on the quantity of output, they struggle with transparency and consistency, often misleading users with their seemingly authoritative grasp of language. Imagine delving into the world of clinical terms, only to be met with “hallucinations”—those moments when an AI confidently conjures up a plausible-sounding yet utterly erroneous response.
The Illusion of LLMs
LLMs wield a fascinating capability, crafting meaningful and context-aware language across substantial passages. They serve as the backbone for translation tools, content generators, and even automated customer service agents, outperforming traditional ML in tasks such as entity recognition and classification.
However, these models are not without their flaws. With soaring computational costs and unpredictable outputs, LLMs face a rocky road, riddled with accuracy concerns and regulatory challenges. Consider a scenario where a user queries ChatGPT for the MedDRA code pertaining to a “heart attack.” While the LLM delivers what appears to be an authoritative response, it’s tragically several steps away from the truth—offering the code for mesothelioma instead.
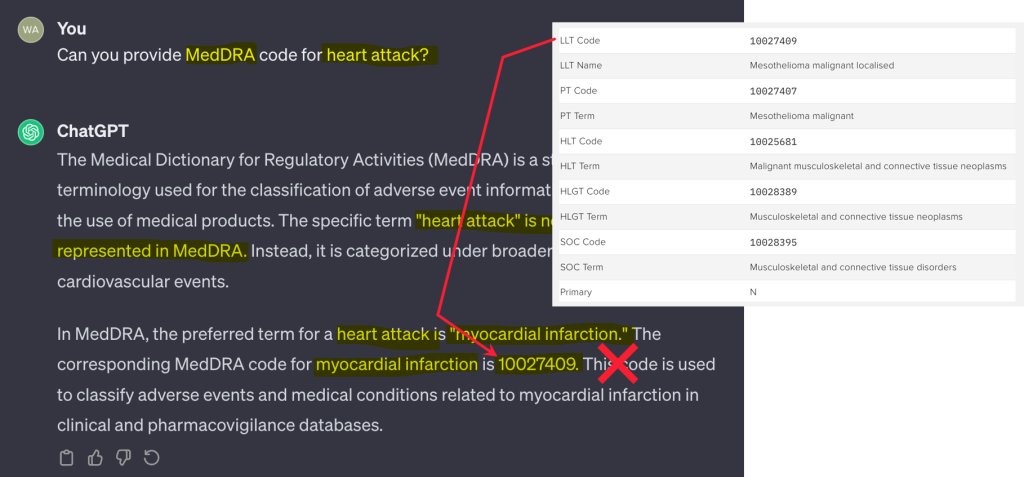
This deception isn’t born from malice but rather from the model’s mechanics—selecting the most statistically probable word without the necessary grounding in factual accuracy. Thus, any endeavor to sculpt reliable outputs must demand scrutiny. Even a layer of human verification may falter, as LLMs can project such an air of credibility that discerning fact from fiction becomes a daunting task.
Contrarily, supervised machine learning models emerge with a distinct advantage. Their presumed unreliability strips away the persuasive narratives that confuse users, fostering a relationship based on familiarity and trust. In the context of clinical research, where precision is paramount, this foundation becomes essential.
The Uphill Battle of Supervised Machine Learning
In the realm of clinical trials, issues around data privacy converge with the need for transparency, making the case against the wholesale adoption of LLMs clear. Supervised ML systems often operate at a fraction of the cost associated with LLMs, frequently delivering superior scalability, effectiveness, and maintainability.
Industry frontrunners like Zelta, developed by Merative, advocate a philosophy centered on deploying the right tool for the right task, prioritizing customer value in AI-driven clinical research. The siren call of LLMs can be seductive, but in seeking the optimal path, companies may find that the straightforward efficiency and concrete returns of supervised ML are not just appealing— they’re essential.
Whereas LLMs have dazzled with their linguistic acumen, Zelta’s experiences underscore the pragmatic advantages of supervised ML. By retaining greater control over data, these models ensure adherence to accuracy and compliance standards, thus minimizing unpredictable results and maximizing actionable insights. In a world where precision can pivot an entire trial’s outcome, the meticulous oversight offered by supervised models becomes a vital asset.
For specific clinical trial requirements, the bespoke nature of supervised ML shines through, tailored to fit unique data conditions rather than forcing a one-size-fits-all AI solution. With the potential burden of extensive training data alleviated, these models can be embedded into workflows, fostering a productive feedback loop with human operators.
Although acquiring the necessary training data can be a formidable task—often both labor-intensive and costly—supervised ML promises substantial long-term savings and efficiency. When harnessed judiciously, these models can morph enduring data hurdles into innovative strategic advantages.
Zelta: Pioneering AI in Clinical Trials
For over a decade, Zelta, an industry pioneer, has woven AI and advanced automation into the fabric of clinical trial management. By leveraging a cloud-based eClinical platform, the complexities of managing clinical trials have been simplified, buoyed by cutting-edge AI tools for medical coding and CDASH annotation. This marriage of technology has culminated in substantial labor savings and enhancements in both accuracy and efficiency across trials.
As AI’s influence in clinical research continues to expand, Zelta remains steadfast, vowing to enhance its AI capabilities based on proven use cases rather than succumbing to fleeting trends. This discerning approach isn’t merely cautious; it is a commitment to genuine innovation and the delivery of tangible results in the field of clinical studies.